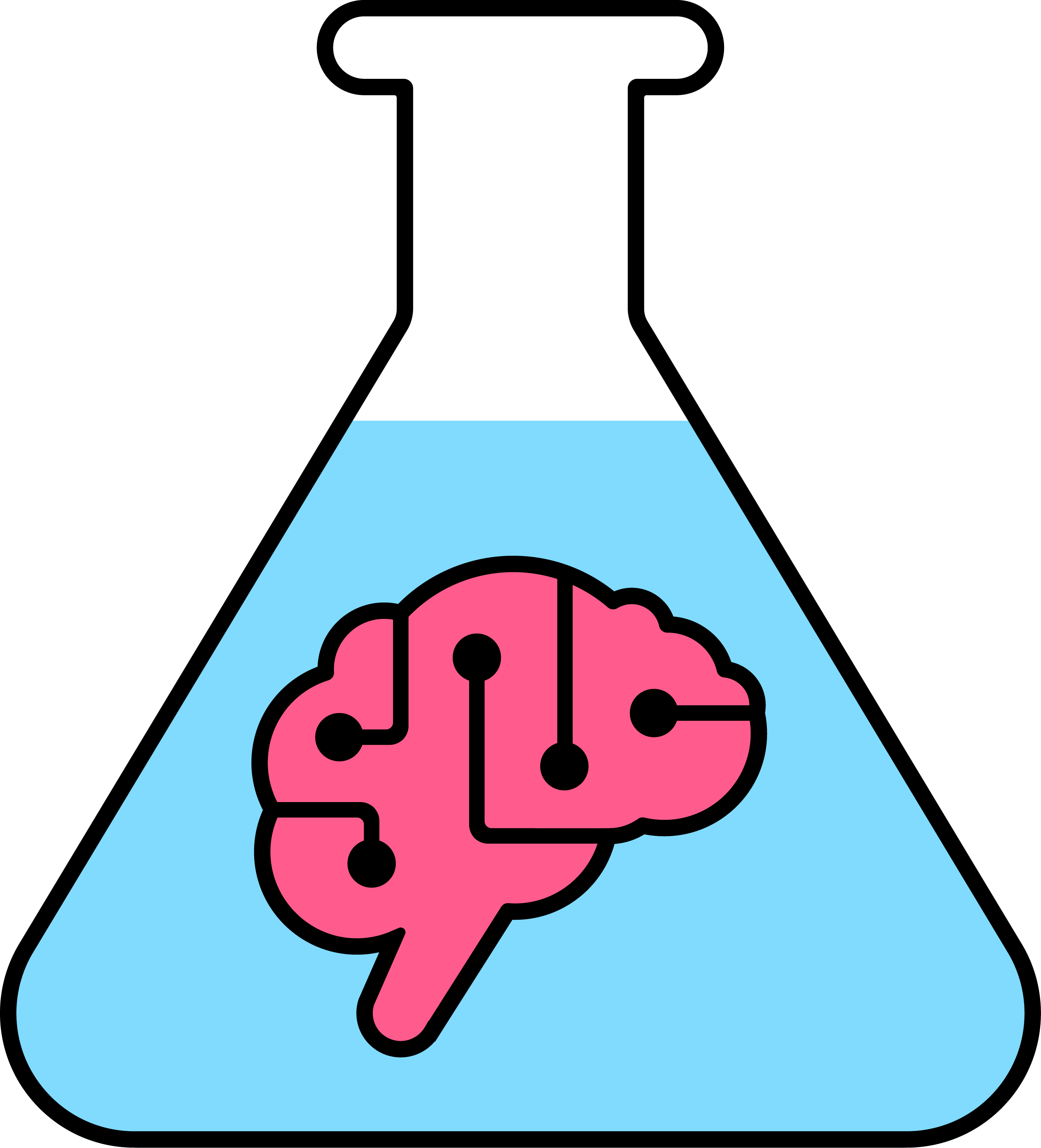
Nabler is now Brainlabs
In a match made in marketing heaven, Nabler has become part of Brainlabs, the global, high performance media agency.
This means we’ve combined our powerful Data Science, Data Engineering, CRO & Digital Analytics expertise with Planning, Media, Tech, Creative and more!